There are several normality tests: Shapiro-Wilk, Kolmogorov-Smirnov, Anderson-Darling, Liliefors, Jarque-Bera, Anscombe-Glynn, Cramer–von Mises, etc.
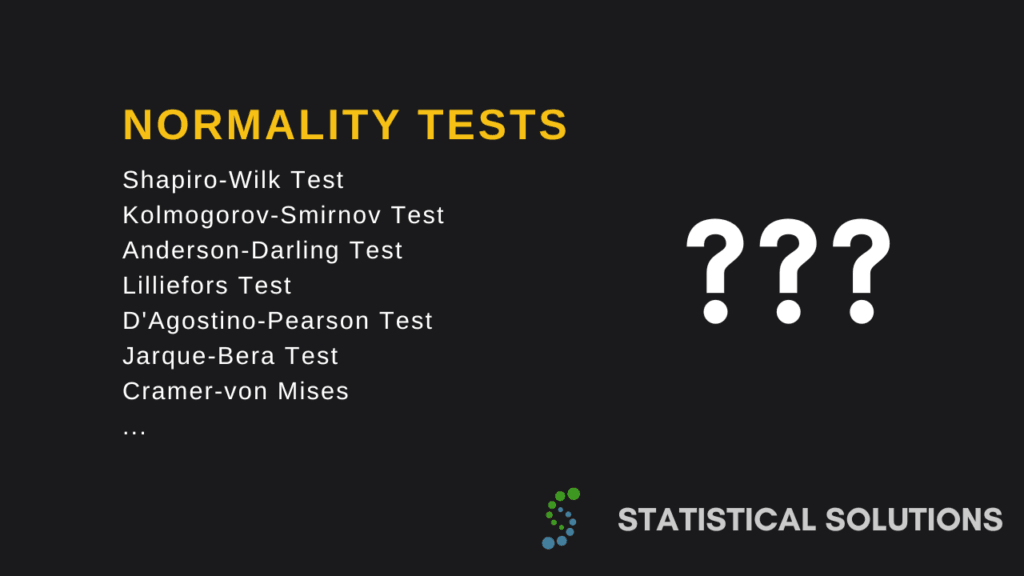
Pitfalls
♦️ “Non-” Normality tests” vary in the type of departures from normality they can detect and therefore it is not unlikely that they may CONTRADICT each other on the basis of their p-values.
♦️ Practically speaking, you may conclude that the data come from a Normal distribution with test A and conclude otherwise with test B.
♦️ Using a single test, say Shapiro-Wilk results in investigating a single angle in which data may deviate from normality and it may be very misleading.
♦️ Furthermore, such tests are sensitive to sample size :
– Large samples will invariably reject the null hypothesis, H0: data come from a Normal distribution
– Small samples are usually not powered to reject the null hypothesis H0
☣️ ☣️ ☣️ Remember that studies are NOT powered for “non-” normality tests!
Good Practices
- The best way to assess non-normality is through visual inspection.
- I suggest the use of a quantile-quantile plot — Q-Q plot and density plots.
- Even better use robust methods.
- When in doubt, it is a good idea to run a sensitivity analysis using a robust method or a permutation test.