Training in biostatistics is highly valuable for life scientists, as it equips them with the necessary tools to design experiments, analyse data, and draw meaningful conclusions in research.
Training in biostatistics for medical science liaisons and medical writers is essential for effectively communicating scientific information, understanding clinical data, and providing valuable insights to healthcare professionals. We have developed a tailored approach for MSLs and medical writers seeking to enhance their biostatistics skills.Fundamentals Statistical Tools
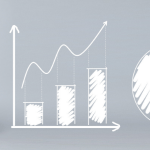
An easy and applied introduction to key statistical concepts. Learn about data visualisation & outlier detection. Demystify statistical significance testing – p-values, significance, power, confidence, etc. An excellent introductory module and a solid basis for all other courses.
Biostats in the Medical Literature
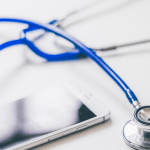
Learn key biostatistical concepts to review & interpret findings published in the medical literature. Selected scientific publications are reviewed, discussed and criticised in terms of bias, uncertainty & scope.
Introduction to the Design of Experiments ‘DOE’
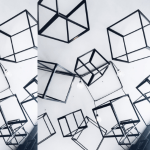
Variation is present in every experiment. Learn about DoE techniques to control variation, and to maximise data quality. Commonly used experimental designs are discussed as well as corresponding statistical data analysis tools.
Advanced Experimental Designs
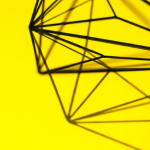
Learn about advanced experimental designs to account for constraints: time, resources, material heterogeneity, randomisation restrictions as well as repeated measures. The construction of advanced designs and their analysis is covered.
Screening Techniques in DOE
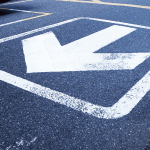
In preliminary research phases, the number of potentially influential factors to investigate is usually large. Screening designs are essential to identify the most influential factors with a reasonable number of runs in preliminary research phases.
Optimisation Designs
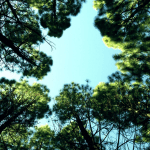
Once influential factors are identified, the next goal consists of optimising their settings. This module covers the construction of experimental designs for optimisation. Data modelling is carried out with response surface methodology.
Statistical Analysis of Metagenomic Data
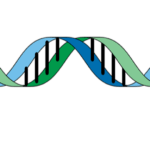
Metagenomic data possess specific features that call for adapted statistical methods. Learn about the most commonly used statistical tools in this field.
Shelf-Life & Stability Studies – Design & Analysis
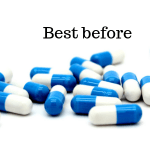
Shelf-life data possess specific features so that their design and analysis require adapted statistical tools. Key elements in the study design are presented and survival techniques used to analyse life data are covered and illustrated.
Linear Regression Modelling Techniques
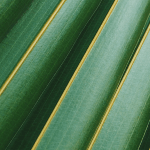
Building a regression model with stats packages has become straightforward. However, interpreting the software output and building a good are no simple tasks. Learn the essentials of model-building, goodness-of-fit tools & common pitfalls.
Principal Component Analysis
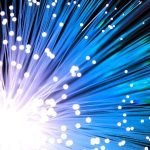
Learn about Principal Component Analysis, a data reduction technique, to identify, quantify & visualise the structure of a set of measurements. PCA provides insightful data visualisation tools. Learn about innovative applications.
Cluster Analysis
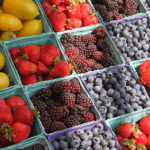
Learn how to take data (consumers, genes, …) and organise them into homogeneous groups for use in many applications, such as market analysis and biomedical data analysis, or as a pre-processing step for many data mining tasks. Learn about this very active field of research in statistics and data mining, and discover new techniques.
Regression Modelling Techniques for Categorical Data
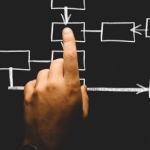
Linear regression is inappropriate to model binary responses such as pass/fail, alive/dead. Learn the principle of logistic regression, its similarities with linear regression and its specific tools. Good practices for model-building are presented.