Engineering Applications of Machine Learning
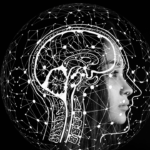
Artificial Intelligence (AI) has become a prominent sale argument no matter whether you want to buy a simple toaster or an advanced assembly line robot. The purpose of this seminar is to demystify the jargon used and to provide attendees with the tools …
Fundamentals Statistical Tools
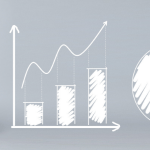
An easy and applied introduction to key statistical concepts. Learn about data visualisation & outlier detection. Demystify statistical significance testing – p-values, significance, power, confidence, etc. An excellent introductory module and a solid basis for all other courses.
Introduction to the Design of Experiments ‘DOE’
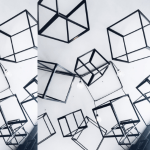
Variation is present in every experiment. Learn about DoE techniques to control variation, and to maximise data quality. Commonly used experimental designs are discussed as well as corresponding statistical data analysis tools.
Advanced Experimental Designs
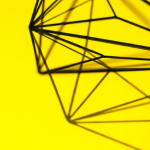
Learn about advanced experimental designs to account for constraints: time, resources, material heterogeneity, randomisation restrictions as well as repeated measures. The construction of advanced designs and their analysis is covered.
Screening Techniques in DOE
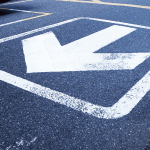
In preliminary research phases, the number of potentially influential factors to investigate is usually large. Screening designs are essential to identify the most influential factors with a reasonable number of runs in preliminary research phases.
Optimisation Designs
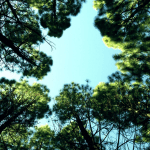
Once influential factors are identified, the next goal consists of optimising their settings. This module covers the construction of experimental designs for optimisation. Data modelling is carried out with response surface methodology.
Statistical Methods for Reliability Studies
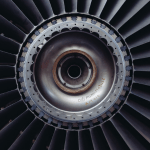
In industrial applications, reliability is crucial and testing is expensive. Collected data must be exploited in the best way possible. Reliability data possess specific features that call for dedicated statistical methods. Learn about statistical tools for reliability analysis.
Linear Regression Modelling Techniques
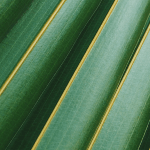
Building a regression model with stats packages has become straightforward. However, interpreting the software output and building a good are no simple tasks. Learn the essentials of model-building, goodness-of-fit tools & common pitfalls.
Principal Component Analysis
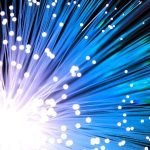
Learn about Principal Component Analysis, a data reduction technique, to identify, quantify & visualise the structure of a set of measurements. PCA provides insightful data visualisation tools. Learn about innovative applications.
Cluster Analysis
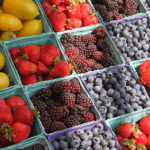
Learn how to take data (consumers, genes, …) and organise them into homogeneous groups for use in many applications, such as market analysis and biomedical data analysis, or as a pre-processing step for many data mining tasks. Learn about this very active field of research in statistics and data mining, and discover new techniques.
Regression Modelling Techniques for Categorical Data
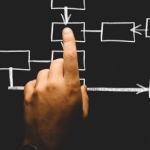
Linear regression is inappropriate to model binary responses such as pass/fail, alive/dead. Learn the principle of logistic regression, its similarities with linear regression and its specific tools. Good practices for model-building are presented.