“Statistical power analysis provides the conventional approach to assess error rates when designing a research study. However, power analysis is flawed in that a narrow emphasis on statistical significance is placed as the primary focus of study design. In noisy, small-sample settings, statistically significant results can often be misleading. To help researchers address this problem in the context of their own studies, we recommend design calculations in which
(a) the probability of an estimate being in the wrong direction (Type S [sign] error)
and
(b) the factor by which the magnitude of an effect might be overestimated (Type M [magnitude] error or exaggeration ratio) are estimated.
We illustrate with examples from recent published research and discuss the largest challenge in a design calculation: coming up with reasonable estimates of plausible effect sizes based on external information.” Gelman et al., 2014
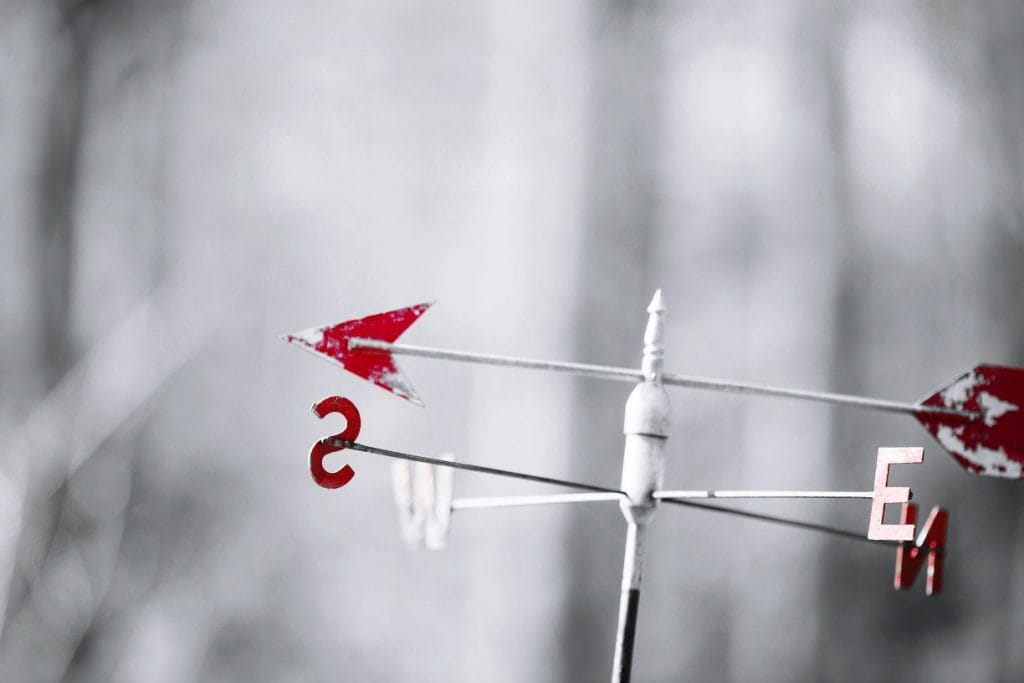
Introduction
This article discusses the potential for significant results to be in the wrong direction and overestimate the true effect when researchers use small samples and noisy measurements in studies. It highlights the importance of power analysis and design analysis, which focuses on estimates and uncertainties rather than statistical significance, to ensure accurate interpretation of findings.
The power of a statistical test is the probability of rejecting the null hypothesis, and depends on sample size, measurement variance, comparisons, and effect size. Power calculations are conditional on these assumptions, including measurement error size, which is typically easier to assess with available data.
The article recommends using statistical calculations based on prior guesses of effect sizes to inform study design, suggesting they should be performed after data collection and analysis. The calculations are framed in terms of Type S and Type M errors, addressing the probability of incorrect sign or magnitude.
Design calculations should use realistic external estimates of effect sizes, as overestimating effects from current study data or isolated literature reports is common practice.
The idea that published effect-size estimates are too large due to publication bias is not new. A method to apply this to specific studies is provided, illustrating with recent studies in biology and psychology. Realistic design analysis suggests larger sample sizes than commonly used in psychology, as a small sample size may lead to a loss in the form of a claim that does not replicate.
Conventional Design or Power Calculations and the Effect-Size Assumption
The postulated effect size is the starting point for design calculations, as the true effect size is unknown. Researchers should have a clear idea of the population of interest and use two standard approaches: empirical, assuming an effect size from previous studies or data, and on the basis of goals, assuming an effect size deemed substantively important.
Conventional approaches to study design can lead to small studies or misinterpreted findings. Preliminary data-based effect-size estimates can be misleading due to selection biases and chance. Determining power under “minimal substantive importance” can also result in larger effect sizes than the true effect.
Statistical authorities often advise against performing power calculations after data collection, as they can overestimate effect size and use post-hoc power analysis as an excuse to explain away nonsignificant findings. However, retrospective design analysis can be useful when strong statistically significant evidence for nonnull effects is found. This approach focuses on the sign and direction of effects rather than statistical significance and uses an effect size determined from external information.
Our Recommended Approach to Design Analysis
A study yields an estimate d with standard error s, which is considered statistically significant if p <.05 and inconclusive otherwise. The next step is to consider a true effect-size D, hypothesized based on external information, and define the random variable drep as the estimate observed in a hypothetical replication study with the same design.
The analysis introduces the hypothetical drep, a conceptual leap that allows for general statements about study design without relying on noisy point estimates. This helps interpret results, revealing the true effect size and information from a given design and sample size.
The probability model for drep considers three key summaries: power, Type S error rate, and exaggeration ratio.
- Power represents the probability of replication drep being larger than critical value
- Type S error rate indicates the probability of incorrect sign
- and exaggeration ratio represents expected Type M error.
The R function, retrodesign(), calculates the true effect size, standard error, statistical significance threshold, and degrees of freedom, generating outputs like power, Type S error rate, and exaggeration ratio, assuming a t-sampling distribution.
Conclusions
Design calculations in null hypothesis test statistics require careful examination of assumptions. A tool has been developed to perform design analysis based on study information and hypothesized population difference or effect size. The goal is not to provide a routine tool but to demonstrate the possibility of such calculations and help researchers understand Type S and Type M errors in realistic data settings.
The article emphasizes the importance of using realistic, externally based estimates of true effect size in power/design calculations, as many investigators use unreliable early data or minimal substantively important concepts, leading to unrealistically large effect-size estimates, especially in environments with multiple comparisons or researcher dfs.
Design calculations assume an effect size and do not add to existing data analysis. However, when used as a tool to use prior information and consider likely direction and size of estimate, they can clarify the true value of a study’s data. Retrospective design calculations may be more relevant for statistically significant findings.
The authors recommend design calculations to address the dangers of erroneous findings in small studies, focusing on the true effect size and variability of estimation. They argue that “significant” findings from underpowered studies can produce wrong answers. Critics have long criticized the lack of attention to statistical power in behavioural sciences, with evidence showing that too many small studies are published when “significant.”
Insufficient attention to issues leads to excessive publication of small studies, often assuming low power statistical significance signifies scientific success under challenging conditions.
The authors argue that statistical significance is not a goal in itself, but rather a tool for scientific understanding. They suggest that confusion about statistical power contributes to the current crisis of criticism and replication in social science and public health research.
References
Gelman A, Carlin J. Beyond Power Calculations: Assessing Type S (Sign) and Type M (Magnitude) Errors. Perspect Psychol Sci. 2014 Nov;9(6):641-51. doi: 10.1177/1745691614551642. PMID: 26186114.