Creascience has been involved in data science since this field of knowledge started gaining traction and has in the maintime developed a strong expertise in statistical support for engineering applications. The combination of both these skills has therefore naturally found its place in our portfolio of solutions.
Our offerings in this field range from an introductory seminar to the use of artificial intelligence and machine learning in engineering to more advanced training options and implementation of custom solutions.
Classical Statistical Support for Engineering R&D
Engineering applications are complex and several factors are likely to have an impact on most processes. Engineers need to know which factors are influential in their applications. In order to manufacture robust products, they also need to evaluate how important they are and optimize their settings.
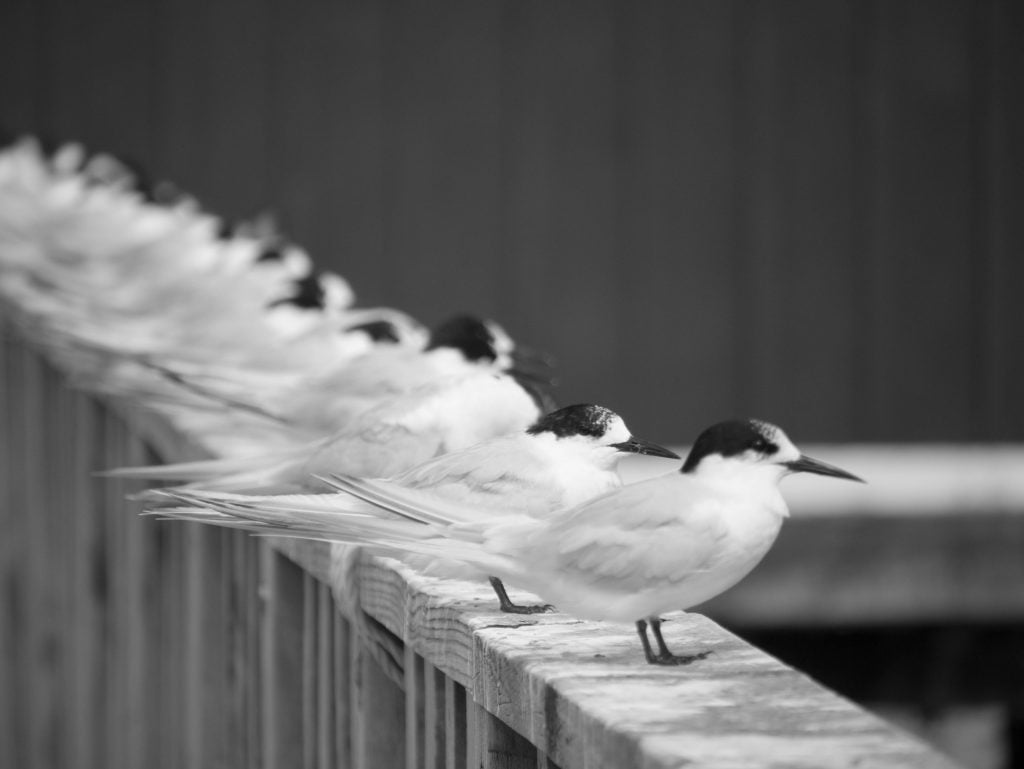
Statistical methods have offered for many years a set of tools designed to control and quantify variation. These methods address two complementary tasks. First, they allow designing efficient experiments. Second, they provide efficient tools for analyzing the experimental data. Even though the dramatic increase in computing power has allowed the development of many new ways of dealing with constraints implied by the original tools, these original methods remain useful and need to be mastered in order to implement meaningful strategies.
Screening & Optimisation Experimental Designs
In order to design experiments efficiently, a wide array of experimental designs is made available to engineers, often referred to as DoE methodology. The choice of the most efficient experimental design depends on the goal of the experiment. Some designs are designed for identifying influential factors, others are designed to optimise factor settings, other are used whenever factors are constrained.
Controlling & Quantifying Variation in the Data Analysis Phase
Statistical methods offer a wide range of tools for controlling, visualising and quantifying variation in experimental data. These methods allow engineers to understand key interactions in processes.
- Data visualisation tools: advanced plots, multivariate data analysis…
- Analysis of designed experiments: ANOVA, ANCOVA…
- Classic modelling techniques: multiple linear regression, nonlinear regression…
- Reliability Analysis – modelling time to failure data: parametric and nonparametric survival analysis…
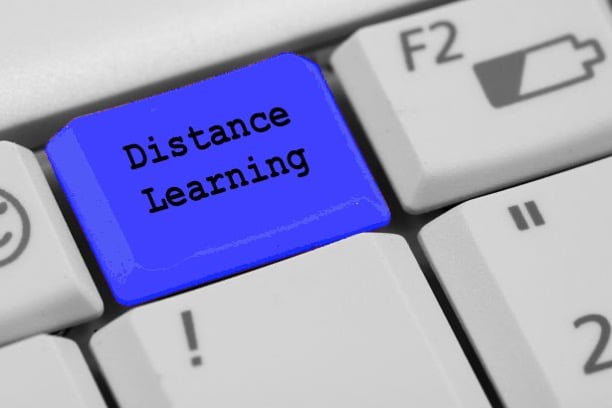
Corporate Training In Engineering Statistics
Looking to improve your self-sufficiency in statistics?
We have been offering customized corporate training in the field of statistics for engineers for more than 25 years. We now focus on live online classes for groups within the same company. You can find details on our most popular topics for engineers here : DOE, regression, reliability, etc.
Advances in Data Science & Engineering Applications
The role of statistics in engineering used to be rather predictable, but the situation has changed in recent years due to the advancement of computer technology and the resulting computing power.
Such advances now enable engineers to harness the power of advanced DoE as well as machine learning tools as a an alternative modelling tool for capturing and predicting complex relationships in factors.
On top of that, these new techniques also allow the analysis of larger sources consisting of less-structured data, like historical databases or real-time evaluation of production data.
Advanced, Dynamic & Flexible Experimental Designs
There are experimental situations where constraints limit the use of classic experimental designs. For instance, combinations of factors at given levels may be intractable. Also, experimental runs may be costly so that the number of runs required by a classical design becomes economically unfeasible.
Several new more adapted techniques like optimal designs and sequential dynamic designs have become available in recent years. These methods use numerical optimization to overcome the often perceived rigidity of classical methods. They enable engineers to maximize the coverage of the experimental domain with a pre-defined number of runs while accounting for any type of constraint they may face.
So on top of being mathematically more efficient than classical methods, these new approaches to experimental design are now considered as the most cost-effective.
Machine Learning as an Alternative Modelling Tool
For many years, modelling of the results of experiments was based mostly for practical reasons on the assumption that the effect of factors on the response was linear. Even though this assumption might seem reasonable on a small experimental domain, it was used in most situations due to limited alternatives. Classic linear models are also not flexible enough and often fail at capturing some important relationships and complex interactions between factors.
The development of machine learning algorithms has led to the implementation of totally new paradigms in the analysis of data where the exact shape of the relationship between an observed response and a variety of factors can be kept very general without losing the capability to understand the nature and magnitude of such relationships.
Such algorithms can be implemented in the context of engineering applications with the advantage of increasing the chance of detecting existing effects of a large number of factors without requiring any additional cost in the experiment.
Machine Learning and Large Process Databases
Machine-learning tools offer an array of much more flexible models. Combined with dramatically increased computing capabilities, they allow novel exploration of existing data, either historical process measures or series of past R&D experiments. Such investigations often lead to discoveries at a highly reduced cost. Furthermore, the more recent modelling tools overcome a frequent criticism of the early developments in this field, namely the “black box” aspect: many recent algorithms allow a clear interpretation of the drivers of a given phenomenon.
Last but not least, these methods may also be implemented for real-time analysis of complex and detailed process data in order to improve QC efficiency and anticipate drifts or failures in a much more efficient way than traditional methods.
Our experts offer state-of-the-art quantitative tools to implement advanced machine-learning based solutions for complex engineering applications.
To learn more: