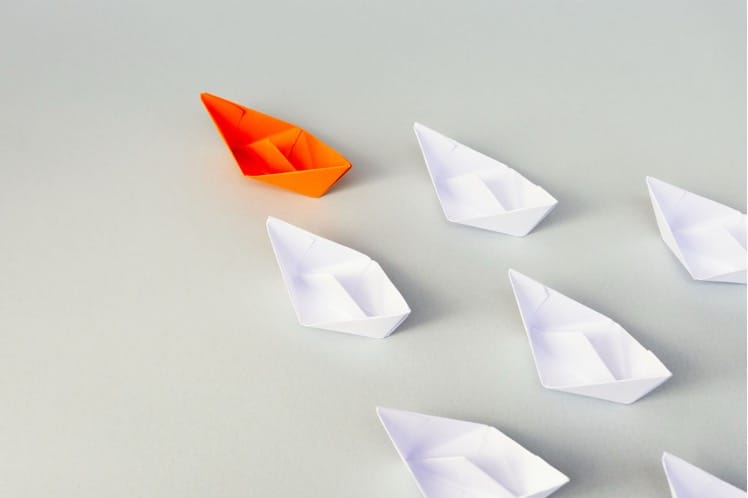
Empowering Biotech Innovation Through Biostatistical Mentoring
Context
For over 25 years, Creascience’s biostatisticians have been collaborating with both young startups and established pharmaceutical and biotechnology companies to train their scientific staff and assist them in setting up, analyzing and interpreting and reporting scientific studies. Building on this experience, we are proud to present a new mentoring program aimed at increasing the autonomy of research teams within biotechnology startups with regard to good statistical and scientific practices.
Scientific research is an essential and critical activity for any startup in the field of life sciences, regardless of its stage of development. What is more, the sustainability of startups in this field depends directly on the quality of this research, whether directly to ensure the validity of the results obtained or to convince potential investors. Finally, the outcome and ultimate success inevitably involve confronting regulatory authorities at one point or another.
All these aspects make the use of good scientific practices and the ability to convince external stakeholders of them essential. In this context, statistical methods – which go well beyond the analysis of experimental data – constitute essential tools, as demonstrated in particular by their omnipresence in the standards of institutions in the health field.
For example…
- USA : The FDA requires that nonclinical trials follow a formal, detailed experimental design that includes steps taken to reduce bias.
- UK : The MHRA produces a specific guide for medical devices in particular to supervise the statistical aspects of R&D.
- Europe: EMA produces specific guidance to support the management of missing data: from trial planning to data analysis
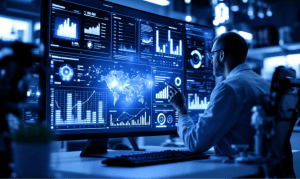
Why Biostatistics Mentoring Matters
The benefits of using rigorous statistical methods are numerous. These include:
Tailoring Mentorship to Your Development Stage
Tailoring a mentoring program in biostatistics to the development stage of biotech companies is important for several reasons. Development stage biotech companies have specific needs and challenges that differ from more established companies, and customizing mentorship can better address these needs.
Discovery Phase
Focus on exploratory data analysis, hypothesis generation, and early experimental design. Statistical mentoring emphasizes efficiency in screening approaches and identifying promising candidates while maintaining scientific rigor despite small sample sizes.
Preclinical Development
Statistical guidance shifts to robust study design, appropriate animal model selection, and developing analysis plans that will satisfy regulatory requirements. Mentors help establish quality control procedures and data management practices essential for IND submissions.
Target Audience
Program Objectives
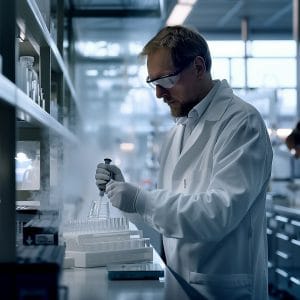
Direct Knowledge Transfer
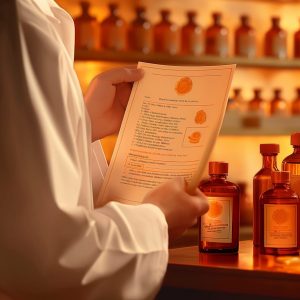
Long-Term Tooting
Biostatistics Mentoring Program Process
As this program represents a long-term commitment, we propose a progressive involvement with the possibility of interrupting the sequence at any time while still deriving notable benefits from the steps already completed.
1. Masterclass: Best Statistical Practices in Life Sciences
First, we offer a 3-hour masterclass presenting the various aspects of good scientific practices in relation to statistical methods.
This masterclass (see attached detailed program) presents examples of both good and bad practices, particularly in relation to regulatory requirements. We recommend that all scientific and managerial staff attend.
Finally, participation in the seminar is a prerequisite for the mentoring program, but it can be offered independently.
2. Practical workshops: 3 serious games to understand the role of statistics in R&D
To raise awareness among research teams about the importance of mastering statistical practices, we offer, after the masterclass, three workshops in the form of cooperative games during which participants are confronted in a playful way with practical situations in which simple statistical tools allow them to solve real-life problems. The topics covered during each session concern, respectively, the management of experiments and collected data, the scientific and financial setup of trials, and finally the interpretation and proper communication of the results obtained.
3. Program Set Up
The program is customized in close collaboration with company management, paying particular attention to the following aspects:
- Selection of the Resources
One or two people are selected to lead the program internally. Their choice and number remain at the client’s discretion. However, we ideally recommend combining one person directly involved in the lab work with another in a position of authority.
- Determining Program Objectives
The action plan described in the following section serves as a basis for discussion.
Ideally, all suggested points should be covered, but depending on the company’s priorities, the weaknesses identified following the initial masterclass, and the budget and time available, it is possible to focus on certain aspects.
To determine the exact objectives, the mentor assigned to the startup meets with representatives of the management team and the selected resource persons and ensures a realistic curriculum and timeline are established, while meeting the expressed needs.
- Determining the Program Duration
The exact duration of the program depends primarily on the objectives set and the anticipated availability of the key stakeholders.
The minimum duration is six months, and ideally one year. However, at the end of the first six-month period, a re-evaluation of the entire process is proposed, and the startup is asked for further involvement on a quarterly basis only.
It is also possible to organize the program over a longer period, either to accommodate limited resource availability or to integrate additional concepts and tools.
4. Program Implementation
Intervention Principle
For each theme:
- Initial meeting – presentation of concepts
- Submission of an analysis grid to transpose the concepts to the company’s reality
- The resources establish a proposed standard
- Second meeting to discuss the proposal
- Adjustments if necessary and final touch to the standard
Mentor Involvement
The mentor is an experienced biostatistician. They share their expertise in two ways:
- Regular meetings with the company’s resource persons, either in person or remotely
- Always available for specific questions by email or telephone
In addition, we have planned several optional components that can be added at any time when the implementation process generates additional unanticipated requests.
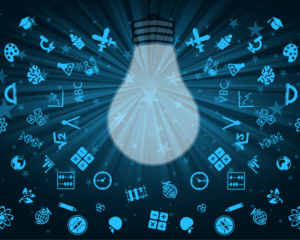
Basic Program Blocks
The ideal program covers all of the following elements, but depending on the needs, it can also focus on certain aspects :
1
Test Data Management & Presentation
- Structure the results of each experiment: adequate formatting, informative dictionary, identification of the nature of each measure
- Take advantage of descriptive statistics: error detection, anticipation of problems
- Ensure the overall integrity of the data: continuity of research.
2
Planning Effective & Useful Tests
- Define the scope of each experiment: target population, number of measurements, primary outcomes, etc.
- Manage bias and variability: replicates vs. repetitions, operator, batch, day effects, etc.
- Determine and justify the size of the trials: statistical significance versus practical/biological significance.
3
Preparation & Management of Laboratory Work
- Write a precise and complete protocol: robustness, transparency and reproducibility
- Manage the progress of experiments: identify anomalies, react to difficulties encountered
- Establish a qualitative assessment: transmit and enrich knowledge of the processes
4
Rigorous Presentation of Results
- Understand the structure of the experiment and perform a data analysis consistent with it
- Adequately interpret the results: p-value, power, equivalence, superiority, non-inferiority
- Write a comprehensive experimental report, a relevant summary, a quality scientific publication
:
Optional Satellite Blocks
Additional needs often arise during these interventions. In order to meet these needs, we offer several blocks that can be integrated into the program.
Common Statistical Challenges in Biotech
Biostatistics plays a critical role in biotech research, but it also faces several challenges. These challenges arise from the unique nature of biotech products, complex data types, and regulatory scrutiny. Here are some common challenges in biostatistics within biotech research.
Small Sample Sizes
Biotech research often involves limited replicates due to cost and ethical considerations.
Our mentors specialize in methods optimized for small samples such as adaptive designs that maximize information from minimal observations while maintaining statistical validity.
Multivariate Data Generation
Modern biotechnology generates vast multivariate datasets through genomics, proteomics, and high-throughput screening.
Mentors may train teams in dimension reduction techniques, multiple testing corrections, and machine learning approaches that extract meaningful signals from complex data.
Handling Missing Data
Biological experiments inevitably generate missing values that can bias results if handled improperly.
Our biostatistical mentors provide expertise in handling missing data so that data integrity and statistical rigor are preserved.
Would you like to find out more ?
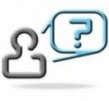