Scientific rigour is a major concern and most scientific journals have a policy on statistical analysis and data representation to ensure that issues of experimental design can be reviewed thoroughly.
Experimental design is one crucial component of a scientific method. “A well-designed, properly conducted experiment aims to control variables in order to isolate and manipulate causal effects and thereby maximize internal validity, support causal
inferences, and guarantee reliable results” Iphofen, R. (Ed.).
When reporting experimental designs in scientific publications, it is essential to include detailed and transparent descriptions to allow for reproducibility and critical assessment.
Today, I decided to present the minimal requirements for reporting experimental design in publications primarily based on the suggestions by Nature – Reporting Life Sciences Research. I have added several points based on over 30 years experience as a biostatistician.
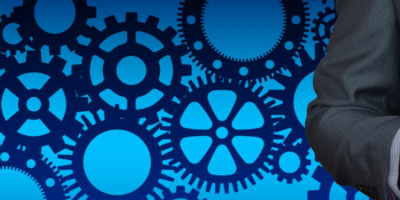
Understanding the structure upon which data are collected
Minimal Requirements for Reporting Experimental Designs
Target Population
- The target population is the group of people or subjects from whom researchers want to draw conclusions, and they will typically select a sample from this population.
- The target population should be clearly defined to ensure that the findings, treatments, or interventions are relevant to the right group. It often includes factors such as age, gender, geographic location, socioeconomic status, health conditions, or other characteristics depending on the context.
Clear Reporting of Inclusion/Exclusion Criteria
- Inclusion criteria are the specific characteristics or conditions that a participant must meet to be eligible for inclusion in a study or experiment. These criteria help define the target population for the study and ensure that participants share certain characteristics relevant to the research question. Inclusion criteria are carefully defined to ensure that the study sample is representative of the population being studied and that the results are generalizable to that population.
- Inclusion criteria are an essential part of study design because they help minimize biases, improve the validity of the results, and ensure the safety and appropriateness of participants for the interventions being tested.
Research Question
- A research question is a clear, focused, and specific question that a study or investigation seeks to answer. It serves as the foundation for the research process, guiding the direction of the study, helping to define the objectives, and determining the methods used to collect and analyze data.
- Research questions help shape the hypothesis (if applicable) and the methodology of the study. They often emerge from a literature review, where existing studies are reviewed to identify gaps or areas that need further exploration.
Outcome Variables
- An outcome variable (also known as a dependent variable or a response) is the variable that a researcher is trying to explain, predict, or measure in an experiment or study.
- It represents the effect or result that is influenced by changes in the independent variable(s) (also known as predictor variables or factors).
- The outcome variable is what you measure in your study to assess the impact of the independent variable(s).
- Identify the primary and secondary outcome variables and why they were chosen.
Experimental Units
- Experimental units refer to the individuals, items, or subjects that are being studied or experimented upon in an experiment.
- They are the basic units of observation or measurement in a study, and the experimental units are assigned to different experimental conditions or treatments.
- In other words, experimental units are the entities that receive the treatment or intervention being tested and for which data is collected.
- Provide a description of the experimental units and the treatments
Sample Size
- Describe how sample size was determined and for which primary outcome
- To do this several pieces of information are required
- Nature of the hypotheses to test (derived from the research question)
- Relevant effect size
- Power to achieve
- Confidence level to achieve
- Nature of the primary endpoint
- Type of experimental design
Effect Size
- Biological/clinical effect size: needed to distinguish statistical significance from biological/clinical relevance.
- It helps to understand whether a particular result is not only statistically significant but also practically or meaningfully important.
Power
- Indicate the power level to achieve
- Power is crucial because it helps to understand whether a study is likely to find meaningful results, especially when those results truly exist. High power means that the test has a high probability of detecting a significant effect, while low power means that the study might fail to detect an effect even if one exists (i.e., a Type II error).
Confidence Level
- Confidence level refers to the degree of certainty or probability that a statistical estimate (such as a confidence interval) contains the true population parameter.
- In simpler terms, it is the percentage of times you would expect the true value of a parameter to fall within the estimated range if you were to repeat the study or sampling process many times.
Experimental Factors and Their Levels
- Experimental groups refer to the groups in a research study or experiment that are exposed to the treatment or condition being tested.
- They are contrasted with control groups, which are not exposed to the experimental treatment but instead receive a baseline or standard treatment for comparison.
- The goal is to determine the effect of the treatment or manipulation by comparing the outcomes between the experimental and control groups.
- Describe whether repeated measures are to be measured.
Type of Factor(s)
- In research and experimentation, factors refer to the variables or conditions that are manipulated, controlled, or measured in a study.
- Depending on the role they play in the experiment, factors can be classified in different ways.
- Crossed
- Each level of one factor is combined with every level of another factor.
- This design allows researchers to examine the interactions between the factors and how they jointly influence the dependent variable.
- Nested
- The levels of one factor are dependent on the levels of another factor, and you do not test all combinations.
- One factor is nested within the levels of the other.
- Fixed
- These are factors that are deliberately controlled or fixed in the design of the study because the researcher is specifically interested in examining them.
- Example: Medication type in a clinical trial
- Random
- These are factors that cannot be controlled or predicted and are often due to inherent variability in populations or experimental conditions.
- Example: Patient, Animal
Nuisance variables
- Variables that can affect the dependent variable or outcome in a research study but are not of primary interest to the researcher. These variables may introduce unwanted variability into the study, making it harder to detect the true effect of the independent variable(s) on the dependent variable.
- While nuisance variables may not be the main focus of the study, they need to be controlled or accounted for in order to avoid confounding the results and to improve the validity of the study’s conclusions.
- Why and how they are accounted for.
Covariates
- A covariate is a variable that is included in a statistical model to account for its potential influence on the outcome (dependent variable).
- Covariates are variables that may affect the dependent variable, but are not the primary focus of the study.
- They are often controlled for in analyses to reduce confounding and improve the precision of the results, allowing researchers to isolate the effect of the independent variable(s) on the dependent variable.
Blinding
- Describe whether the investigators were blinded to group allocation during data collection and/or analysis
- A blinding procedure used to limit the risk of bias in the results
Randomisation
- Describe how experimental units/samples/organisms/participants were allocated into experimental groups
- A randomisation procedure is used to limit the risk of bias in the results
Replication
- Indicate whether true biological replicates or technical replicates were used.
- Several publications fail to clearly report the true nature of the replicates.
- Usually technical replicates are less variable than biological replicates.
- This might lead to a increased risk of false negative and false positive results and obvious reproducibility issues.
Schematic Representation of the Experiment/Trial
- A schematic representation of experimental design visually illustrates how different components of an experiment are organized and how they relate to each other.
- This includes the independent variable(s) (treatment or manipulation), the experimental units (subjects or objects being studied), the control group(s), and the outcome variable(s).
- The diagram often includes arrows, boxes, and labels to indicate the flow of the experiment, from random assignment to treatment conditions, and the process of data collection.
- The Experimental Design Assistant – EDA may be used for this purpose
- https://nc3rs.org.uk/our-portfolio/experimental-design-assistant-eda
- Below, I’ll describe some common types of schematic representations for experimental designs
Type of Experimental Design Used
An experimental design refers to the process of planning an experiment to ensure that the results are valid, reliable, and interpretable.
It includes decisions about how participants or experimental units are selected, how treatments or interventions are assigned, how data are collected, and how the results are analyzed.
The goal of an experimental design is to answer specific research questions while minimizing bias, controlling for confounding variables, and ensuring that the results are attributable to the manipulation of the independent variable(s) rather than external factors.
- Parallel design
- Paired design
- Completely randomized design
- Randomised block design
- Randomised incomplete block design
- Factorial designs
- Cross-over design
- Repeated-measures design
- Split-plot design
- Etc.
Missing Data
Provide the initial n per group and final n per group (explain discrepancies if any).
Conclusion
By including these key elements, the publication will provide a comprehensive and clear account of the experimental design, facilitating reproducibility and critical evaluation by the scientific community.
Some References
- Begley, C. G. & Ellis L. M. 2012. Drug development: Raise standards for preclinical cancer research. Nature, 483, 531-3.
- Butcher NJ, Monsour A, Mew EJ, Chan AW, Moher D, Mayo-Wilson E, Terwee CB, Chee-A-Tow A, Baba A, Gavin F, Grimshaw JM, Kelly LE, Saeed L, Thabane L, Askie L, Smith M, Farid-Kapadia M, Williamson PR, Szatmari P, Tugwell P, Golub RM, Monga S, Vohra S, Marlin S, Ungar WJ, Offringa M. Guidelines for Reporting Outcomes in Trial Protocols: The SPIRIT-Outcomes 2022 Extension. JAMA. 2022;328(23):2345-2356.
- Ionnidis, J. P., Greenland, S., Hlatky, M. A., Khoury, M. J., Macleod M. R., Moher D., Schulz, K. F. & Tibshirani, R. 2014. Increasing value and reducing waste in research design, conduct, and analysis. Lancet, 383, 166-75.
- Iphofen, R. (Ed.). (2020). Handbook of Research Ethics and Scientific Integrity. doi:10.1007/978-3-030-16759-2
- Kilkenny, C., Browne, W. J., Cuthill, I. C., Emerson, M. & Altman, D. G. 2010. Improving bioscience research reporting: the ARRIVE guidelines for reporting animal research. PLoS Biol, 8, e1000412.
- Moher D, Hopewell S, Schulz KF, et al. CONSORT 2010 explanation and elaboration: updated guidelines for reporting parallel group randomised trials. BMJ. 2010;340:c869. doi:10.1136/bmj.c869
- Schulz KF, Altman DG, Moher D; CONSORT Group. CONSORT 2010 statement: updated guidelines for reporting parallel group randomised trials. BMJ. 2010;340:c332. doi:10.1136/bmj.c332