Engineering Applications of Machine Learning
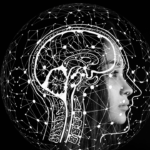
Artificial Intelligence (AI) has become a prominent sale argument no matter whether you want to buy a simple toaster or an advanced assembly line robot. The purpose of this seminar is to demystify the jargon used and to provide attendees with the tools …
Fundamentals Statistical Tools
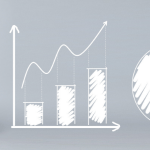
An easy and applied introduction to key statistical concepts. Learn about data visualisation & outlier detection. Demystify statistical significance testing – p-values, significance, power, confidence, etc. An excellent introductory module and a solid basis for all other courses.
Biostats in the Medical Literature
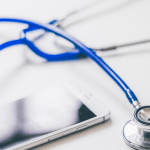
Learn key biostatistical concepts to review & interpret findings published in the medical literature. Selected scientific publications are reviewed, discussed and criticised in terms of bias, uncertainty & scope.
Introduction to the Design of Experiments ‘DOE’
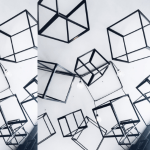
Variation is present in every experiment. Learn about DoE techniques to control variation, and to maximise data quality. Commonly used experimental designs are discussed as well as corresponding statistical data analysis tools.
Advanced Experimental Designs
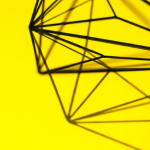
Learn about advanced experimental designs to account for constraints: time, resources, material heterogeneity, randomisation restrictions as well as repeated measures. The construction of advanced designs and their analysis is covered.
Screening Techniques in DOE
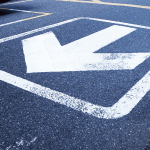
In preliminary research phases, the number of potentially influential factors to investigate is usually large. Screening designs are essential to identify the most influential factors with a reasonable number of runs in preliminary research phases.
Optimisation Designs
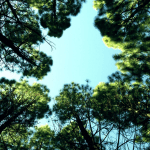
Once influential factors are identified, the next goal consists of optimising their settings. This module covers the construction of experimental designs for optimisation. Data modelling is carried out with response surface methodology.
Statistical Analysis of Metagenomic Data
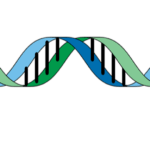
Metagenomic data possess specific features that call for adapted statistical methods. Learn about the most commonly used statistical tools in this field.
Statistical Methods for Reliability Studies
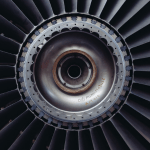
In industrial applications, reliability is crucial and testing is expensive. Collected data must be exploited in the best way possible. Reliability data possess specific features that call for dedicated statistical methods. Learn about statistical tools for reliability analysis.
Shelf-Life & Stability Studies – Design & Analysis
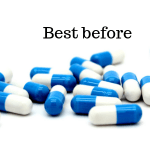
Shelf-life data possess specific features so that their design and analysis require adapted statistical tools. Key elements in the study design are presented and survival techniques used to analyse life data are covered and illustrated.
Linear Regression Modelling Techniques
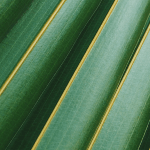
Building a regression model with stats packages has become straightforward. However, interpreting the software output and building a good are no simple tasks. Learn the essentials of model-building, goodness-of-fit tools & common pitfalls.
Multivariate Data Analysis School – 3 days
An applied set of modules with focus on the most widely used multivariate methods and their applications in several fields of application. Learn about the principle of the methods, the data needed, and the information they provide.
Sample Size & Power Determination
Efficient experiments must be large enough to detect meaningful scientific differences and maximize the use of available resources. Learn about sample size and power calculations.
Statistical Comparison of Groups
Several experiments are conducted to determine whether differences exists between procedures, methods, treatments. Learn about the design and the analysis of simple comparative experiments and more complex situations.
Rank-Based Methods
Classical statistical methods such as ANOVA, linear regression rely on certain data distribution assumptions. Whenever they are not met, alternative methods such as nonparametric may be used. Learn about their principle, advantages and limitations.
Repeatability & Reproducibility Studies
The main goal of R&R studies is to determine which sources of variability contribute the most to the overall process variation and how to decide if the measurement system is acceptable or not. Learn how to set up repeatability and reproducibility studies and how to analyse the results with the appropriate statistical methods.
The Virtual Statistician
Through an innovative membership formula, the Virtual Statistician grants access to a unique expertise, renowned internationally, for all projects requiring regular statistical support .Do you wish you had access to the expertise of a statistician?Do y …
A Primer on Predictive Analytics
Predictive analytics (PA) is on everyone’s lips. But what is it really all about? Discover its principle, implementation, typical pitfalls and good practices. Learn about data wrangling and munging, a crucial step in predictive analytics. An overview of the most commonly used models is also presented.
Introduction to R Programming
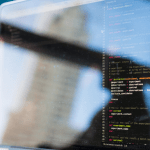
This module offers an easy introduction to R programming. Learn the basics of R programming and the commonly used plots and statistical tools without pain.
Principal Component Analysis
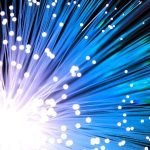
Learn about Principal Component Analysis, a data reduction technique, to identify, quantify & visualise the structure of a set of measurements. PCA provides insightful data visualisation tools. Learn about innovative applications.
Factor Analysis
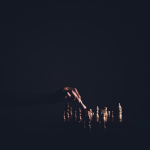
One of the oldest multivariate techniques, factor analysis is closely related to PCA and even confused by many for PCA. However, it serves a totally different purpose. Uncover hidden dimensions in your data.
Correspondence Analysis
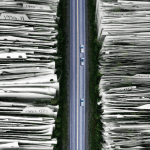
Conceptually similar to PCA, correspondence analysis a method is designed for discovering associations in categorical rather than continuous data. Discover informative 2D-plots for efficient data mapping.
Discriminant Analysis
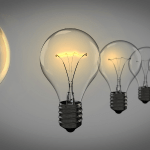
The primary goal of this method is to discover which variables have the best ability of discriminating between two or more known groups in your data. Discriminant analysis may also be used to build predictive analytics models.
Cluster Analysis
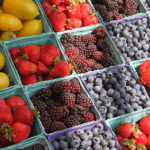
Learn how to take data (consumers, genes, …) and organise them into homogeneous groups for use in many applications, such as market analysis and biomedical data analysis, or as a pre-processing step for many data mining tasks. Learn about this very active field of research in statistics and data mining, and discover new techniques.
Regression Modelling Techniques for Categorical Data
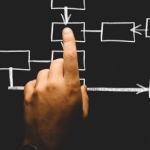
Linear regression is inappropriate to model binary responses such as pass/fail, alive/dead. Learn the principle of logistic regression, its similarities with linear regression and its specific tools. Good practices for model-building are presented.
PLS Regression
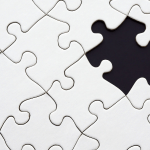
Classical linear regression is inappropriate when predictors are correlated – multicollinearity. Learn about Principal Component Regression (PCR) & Partial Least Squares (PLS) regression to deal with multicollinearity and when it is relevant to use them.
Tree-Based Modeling Techniques
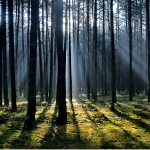
This module offers an overview of tree-based modeling techniques. Learn how they work, when to use them, their strengths and weaknesses, and their implementation including validation. Several real-life applications are presented.
Introduction to Biostatistics
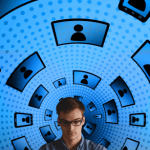
Learn about key biostatistical concepts and efficient tools for summarising & visualising data. Demystify the statistical testing approach used to make decision in the presence of uncertainty.
Fundamental Statistical Tools for Engineers
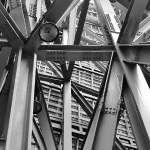
Learn key concepts in statistics. Classical and more recent exploratory data analysis techniques to efficiently summarise data and to detect outliers are covered. Statistical testing and decision-making in the presence of variation are also discussed.